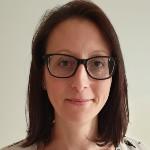
Dr Laura Webber, Chief Operating Officer and Co-Founder of HealthLumen, and Honorary Assistant Professor at the London School of Hygiene and Tropical Medicine, sets out the role of computer modelling in decision making in the post-COVID-19 world.
In the UK, and many other countries, computer simulation models are being used to help predict the spread of COVID-19, and aim to show what sort of government actions could be most effective in managing the outbreak.
Models are valuable tools for planning because, by making certain assumptions, they can examine the impact of different interventions, and therefore identify the optimal measures for mitigating the spread of diseases. In the case of COVID-19, computer modelling aims to quantify the future impact, given different intervention strategies including a ‘no intervention’ baseline scenario i.e. what could potentially happen if governments did nothing. This exercise is key to helping determine which interventions and policies are potentially most effective in ensuring that the health system is appropriately resourced and the disease is eventually controlled.
Understandably there has been considerable debate, and indeed controversy, about the role of modelling in informing these critical decisions, which affect every aspect of people’s lives.
The role of modelling in Public Health decision-making
Computer simulation models are not new. They have been used for many years in a number of fields including:
- Finance - to predict the performance of financial assets and investments
- Climate change - to test hypotheses and draw conclusions about past and future climate systems
- Infectious diseases - to understand the spread of contagious diseases such as HIV, Ebola, and COVID-19
- Non-communicable diseases (NCDs) - to quantify the future social and economic burden of conditions such as heart disease, liver disease and cancer
Common to each of these areas are the simulations of the various ’futures’ that are predicted to occur as a result of different interventions or scenarios. Simulation models use detailed data to make a virtual representation of the population of a specific area, city or country, which then allows for ’virtual experiments’ to determine what the likely outcomes are going to be under different circumstances. For public health models, the virtual world starts with an infection or disease and the model predicts how it will progress.
However, the limitations and effectiveness of any model are dependent on two key aspects: the underlying assumptions, and the input data. Furthermore, there may be limitations on the structure of the model itself, depending on the type of questions it is aiming to address. Many decisions need to be taken about what parameters should be included or excluded. The more variables that are included, the greater the possibility for predicting accurate scenarios, but that also introduces more complexity.
Accurate modelling requires detailed data, and the more robust the data, the more accurate the predictions. With COVID-19 there are still many unknowns on the assumptions. The data was sparse at the start of the epidemic and remains patchy. Other model parameters have had to be entirely assumed – for example, that there is no natural immunity to COVID-19. The less robust the data or the less accurately the model is able to represent the real world, the greater the uncertainty about the predictions.
The role of modelling in the post-COVID-19 world
Despite this uncertainty, use of modelling enables greater foresight than going blind into a decision. This view is best expressed in an old adage, coined by the British statistician George E.P. Box, “All models are wrong, but some are useful”.
So, whilst it is important to acknowledge the limitations of modelling, this does not detract from their critical role in helping to inform overall decision-making processes. All computer simulation models, not just for public health, are a simplification of the real world. They cannot, and never claim to be, complete or 100% accurate. Perfection is not possible and is not the objective. Put simply, the goal is to be more useful than no model.
The role of modelling in the current pandemic has been important in providing evidence to help inform government decisions on a range of policy options and interventions to combat the disease, such as the current policies of social distancing, closing schools and banning mass gatherings, despite the economic consequences.
Beyond COVID-19, we expect to see greater use of modelling by governments, advocacy bodies, and the private sector, to support and enhance their decision-making before expensive and potentially disruptive real-world implementation.